Discover how virtual patients are revolutionizing shared decision-making (SDM) training, offering scalable, cost-effective, and personalized learning solutions for medical professionals.
Introduction
Shared decision-making (SDM) is a collaborative process where clinicians and patients jointly decide on tests, treatments, management strategies, or support packages, based on clinical evidence and the patient’s informed preferences. Despite its significance, SDM is often inadequately taught, particularly at scale. This article delves into the transformative potential of virtual patients in SDM training.
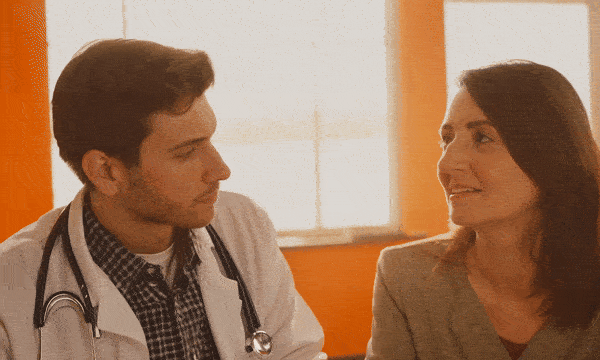
The Challenges of Traditional SDM Training with SPs
Traditional SDM training methods face numerous challenges. Standardized patients (SPs) and role-playing exercises often suffer from inconsistent quality, lack of standardization, and high costs.1-2 Additionally, sourcing sufficient SPs and real patients, particularly in underserved regions and for rare diseases, is problematic. This inconsistency hampers the effectiveness of SDM training.
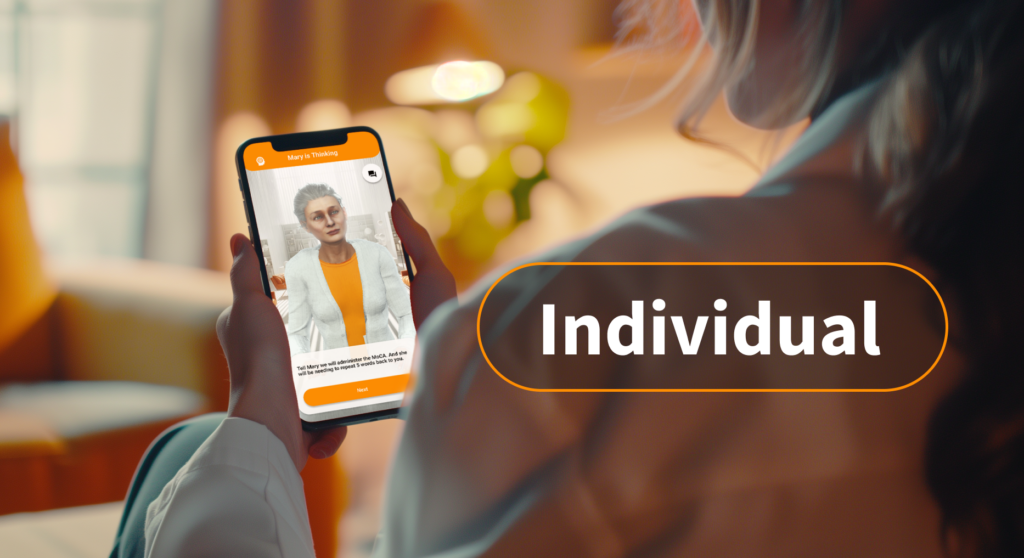
The Importance of Effective SDM Training
Effective SDM training is crucial for improving patient outcomes and enhancing clinician-patient relationships. It empowers patients to make informed decisions and fosters trust. Proper SDM training ensures that clinicians are equipped to facilitate meaningful conversations, leading to better healthcare experiences.
Current State of SDM Training
Currently, SDM training is underrepresented in medical education. In undergraduate medical programs, consultation skills may constitute as little as 0.15% of the curriculum,3 with minimal focus on SDM.4 This lack of formal and systemic training results in uncertainty and variability in SDM application among healthcare providers.5-9
Technological Innovations in Medical Training
The advent of technology in medical education has paved the way for innovative training solutions. Virtual patients, powered by generative artificial intelligence (AI), represent one such advancement, offering interactive and personalized learning experiences.
Introduction to Virtual Patients
Virtual patients are AI-driven simulations designed to mimic real patient interactions. They provide a dynamic platform for clinicians to practice and hone their SDM skills in a controlled, risk-free environment.
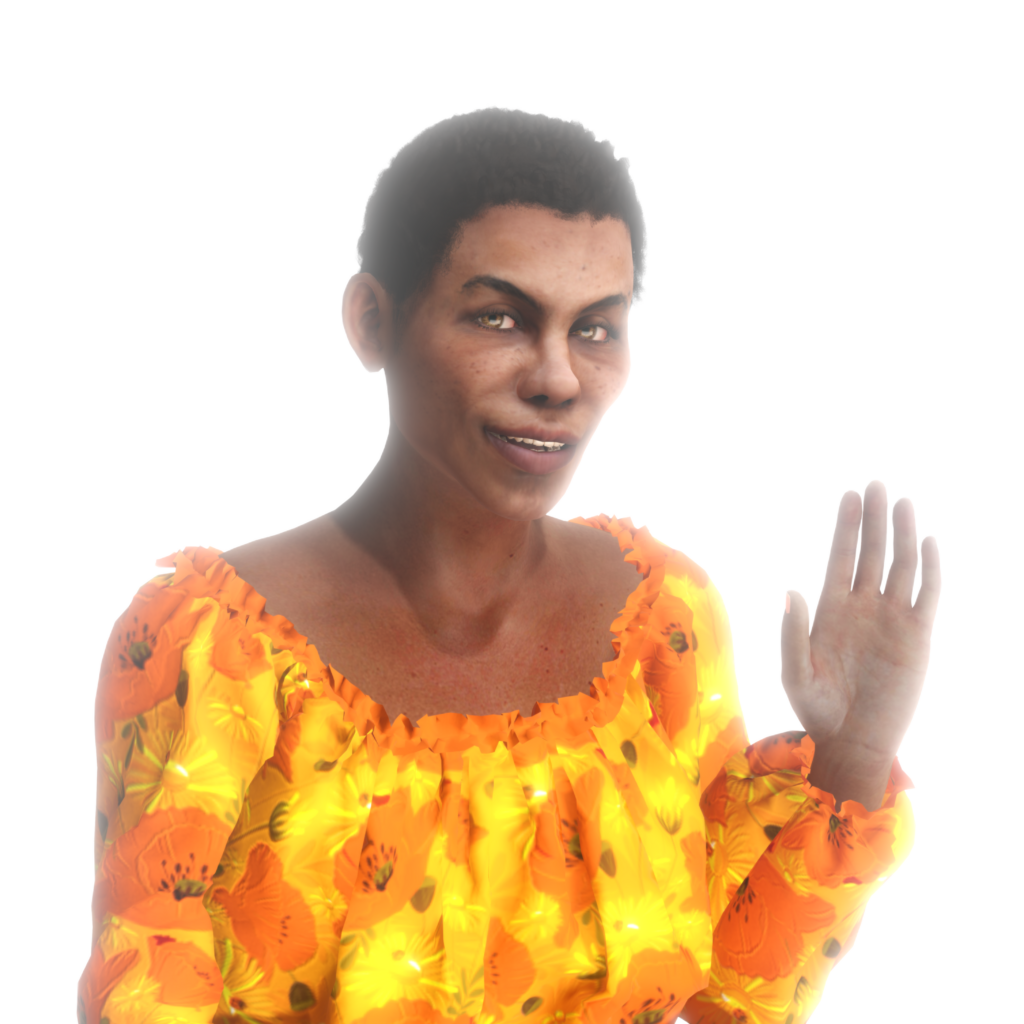
How Virtual Patients Work
Virtual patients leverage sophisticated AI algorithms to simulate realistic patient scenarios. They can respond to clinician inputs, exhibit varied emotional responses, and provide feedback, creating an immersive learning experience. This technology enables repetitive practice, essential for mastering SDM skills.
Advantages of Virtual Patients in SDM Training
Virtual patients offer numerous advantages over traditional training methods. They are cost-effective, scalable, and accessible, allowing for widespread adoption. Personalized feedback from virtual patients enhances learning, and their consistency ensures standardized training across diverse learner groups.
Xuron’s Platform for SDM Training in CME
Xuron’s platform addresses the shortcomings of traditional SDM training by offering a robust, scalable, and effective solution. It integrates virtual patients into various learning modalities, making SDM training more engaging and impactful, especially in CME.
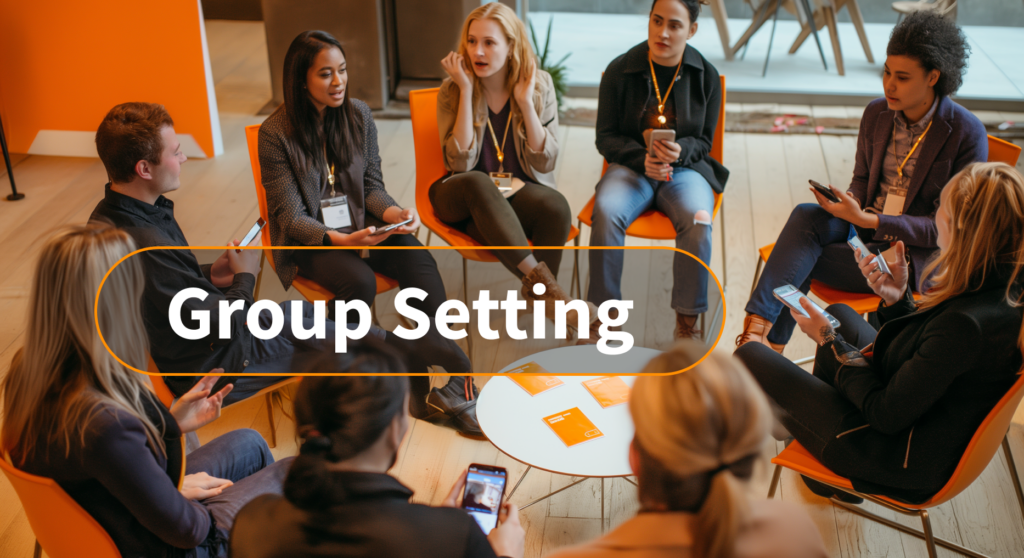
- Interactive Stations at Conferences. At medical conferences, Xuron’s platform allows attendees to interact with virtual patients using their devices. This hands-on experience, tailored to different medical specialties, facilitates practical learning and immediate application of SDM principles.
- Integration into Workshops. AI avatars from Xuron can be incorporated into live workshops, enabling small groups to engage with virtual patients on their phones. This setup fosters interactive discussions and feedback sessions, enhancing the overall learning experience.
- Live Demonstrations in Plenary Sessions. Plenary sessions can feature live interactions between experts and AI avatars, showcasing how to handle complex patient cases in real-time. These demonstrations provide valuable insights into effective SDM practices.
- Recorded Demonstrations and Digital Activities. Traditional digital learning activities can be enriched with recorded sessions where presenters interact with AI avatar patients. These sessions demonstrate key medical concepts and communication techniques, offering a visual and practical guide for learners.
- Needs Assessments Using Virtual Cases: Xuron’s virtual cases can assess learners’ knowledge and skills, identifying areas requiring additional training. This targeted approach ensures that SDM training is tailored to individual needs, enhancing overall competency.
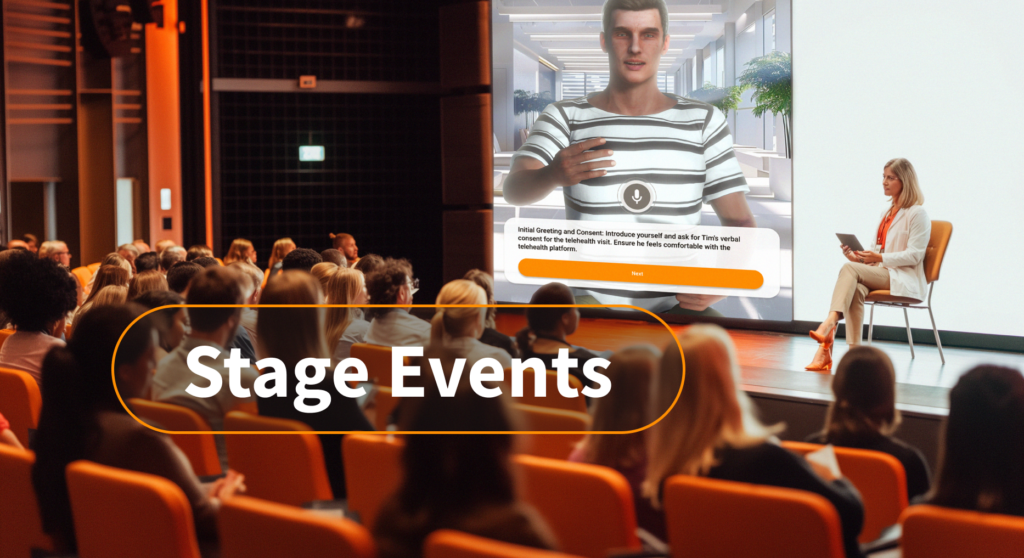
Skill Development with Virtual Patients
Virtual patients are excellent tools for developing crucial skills such as professionalism, communication, clinical decision-making, and motivational interviewing. They provide a safe environment for repeated practice and refinement of these skills.
Scalability and Cost-Effectiveness of Virtual Patients
Compared to traditional methods, virtual patients are more affordable and scalable. They offer personalized learning experiences for every learner, making quality SDM training accessible to a broader audience.
Customizable Content for Specific Needs
Virtual patients can be customized to cover any medical topic or specialty, including rare diseases and underrepresented communities. Their personalities and appearances can be adjusted to create diverse patient profiles, enhancing the realism and relevance of training.
Accessibility and Convenience
Xuron’s platform allows learners to practice anytime, anywhere, using their phones, tablets, or computers. This flexibility ensures continuous learning opportunities, accommodating busy schedules and varied learning environments.
Standardized and Immediate Feedback
One of the key benefits of virtual patients is the provision of standardized, personalized, and immediate feedback. This feedback focuses on clinical skills, communication, and problem-solving, helping learners to improve effectively.
Data-Driven Insights from Virtual Training
The AI in virtual patients analyzes interactions, providing insightful data on learner performance. This data identifies strengths and weaknesses, guiding future training and curriculum development.
Rapid Development of Simulation Cases
Xuron’s platform enables the creation of new simulation cases within just 30 days. This rapid development capability ensures that training content remains current and responsive to emerging medical trends and needs.
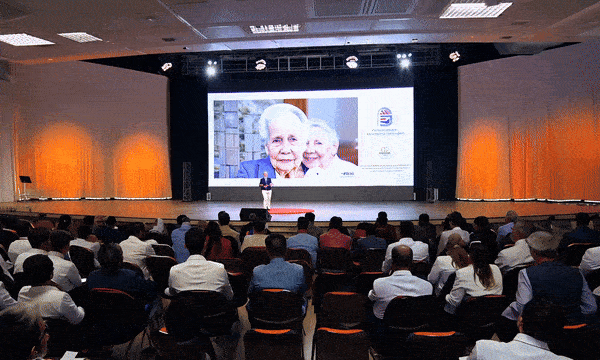
Conclusion
Xuron’s virtual patient platform addresses critical gaps in SDM training by offering a scalable, cost-effective, and impactful solution, especially important in CME. Through interactive stations, live workshops, plenary sessions, recorded demonstrations, and the ability to practice anywhere and anytime, Xuron provides numerous hands-on learning opportunities.
Furthermore, the platform’s accessibility and the insightful data it generates are invaluable for CME educators. As the demand for better SDM training grows, Xuron is at the forefront of transforming how medical professionals acquire and refine these essential skills.
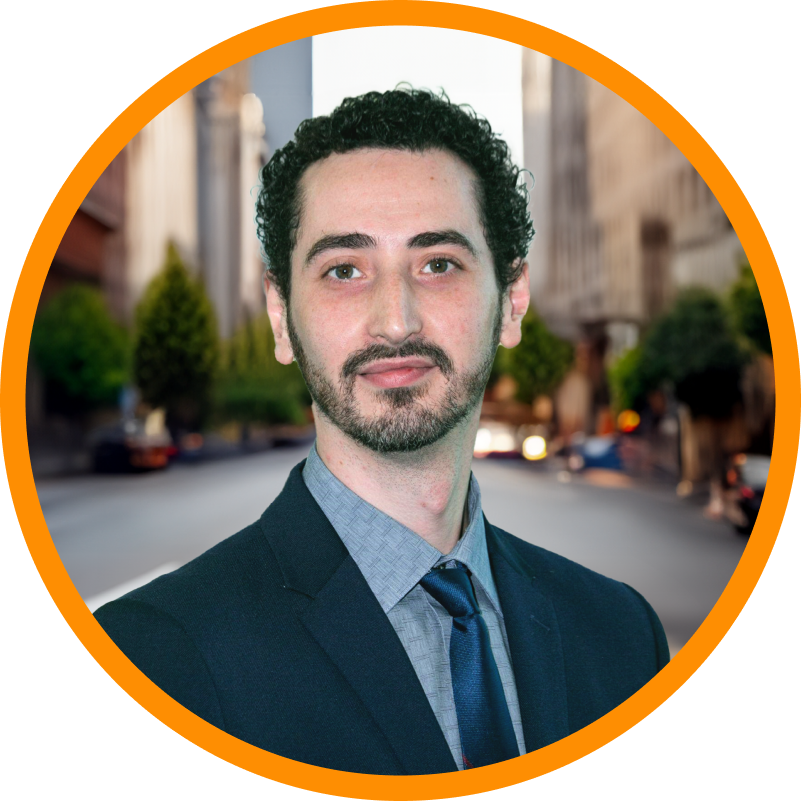
Written By:
DR. BORIS ROZENFELD
Dr. Boris Rozenfeld is a vanguard in medical education, melding his extensive clinical knowledge with a flair for innovative learning design. At the helm of transformation initiatives in healthcare education, His significant contributions include revolutionizing the American Heart Association’s certification programs with adaptive learning technology, significantly boosting knowledge acquisition and practitioner proficiency.
With a career spanning over nine years in continuing medical education (CME), Boris has a rich history with esteemed organizations such as Pri-Med and RMEI, where he created impactful educational programs for both generalists and specialist clinicians. A fervent proponent of generative AI, Boris is at the cutting edge, continuously exploring and implementing new educational designs that integrate large language models.
His dedication to the future of healthcare education is unwavering. Boris is committed to developing sustainable and scalable training solutions that seamlessly marry clinical excellence with contemporary educational strategies.
References
- Smith CM, Gephardt EG, Nestel D. Applying the theory of Stanislavski to simulation: stepping into role. Clin Simul Nurs. 2015 Aug;11(8):361–7. doi: 10.1016/j.ecns.2015.04.001.
- Yardley S, Irvine AW, Lefroy J. Minding the gap between communication skills simulation and authentic experience. Med Educ. 2013 May;47(5):495–510. doi: 10.1111/medu.12146
- Hargie O, Boohan M, McCoy M, Murphy P. Current trends in communication skills training in UK schools of medicine. Med Teach. 2010;32(5):385–91. doi: 10.3109/01421590903394603.
- Durand M, DiMilia PR, Song J, Yen RW, Barr PJ. Shared decision making embedded in the undergraduate medical curriculum: a scoping review. PLoS One. 2018;13(11):e0207012. doi: 10.1371/journal.pone.0207012.
- Allen KM, Dittmann KR, Hutter JA, Chuang C, Donald ML, Enns AL, et al. Implementing a shared decision-making and cognitive strategy-based intervention: knowledge user perspectives and recommendations. J Eval Clin Pract. 2020;26(2 PG-575–581):575–581. doi: 10.1111/jep.13329
- Schoenfeld EM, Goff SL, Elia TR, Khordipour ER, Poronsky KE, Nault KA, Lindenauer PK, Mazor KM. A qualitative analysis of attending physicians’ use of shared decision-making: implications for resident education. J Grad Med Educ. 2018;10(1):43–50. doi: 10.4300/JGME-D-17-00318.1.
- Pyl N, Menard P. Evaluation of nurses’ perceptions on providing patient decision support with cardiopulmonary resuscitation. ISRN Nurs. 2012;2012:591541.
- Giacco D, Mavromara L, Gamblen J, Conneely M, Priebe S. Shared decision-making with involuntary hospital patients: a qualitative study of barriers and facilitators. BJPsych Open. 2018;4(3 PG-113–118):113–118. doi: 10.1192/bjo.2018.6.
- Schoenfeld EM, Goff SL, Elia TR, Khordipour ER, Poronsky KE, Nault KA, et al. Physician-identified barriers to and facilitators of shared decision-making in the emergency department: an exploratory analysis. Emerg Med J. 2019;36(6):346–354. doi: 10.1136/emermed-2018-208242.